Agriculture Dataset for Machine Learning: Unlocking Business Opportunities
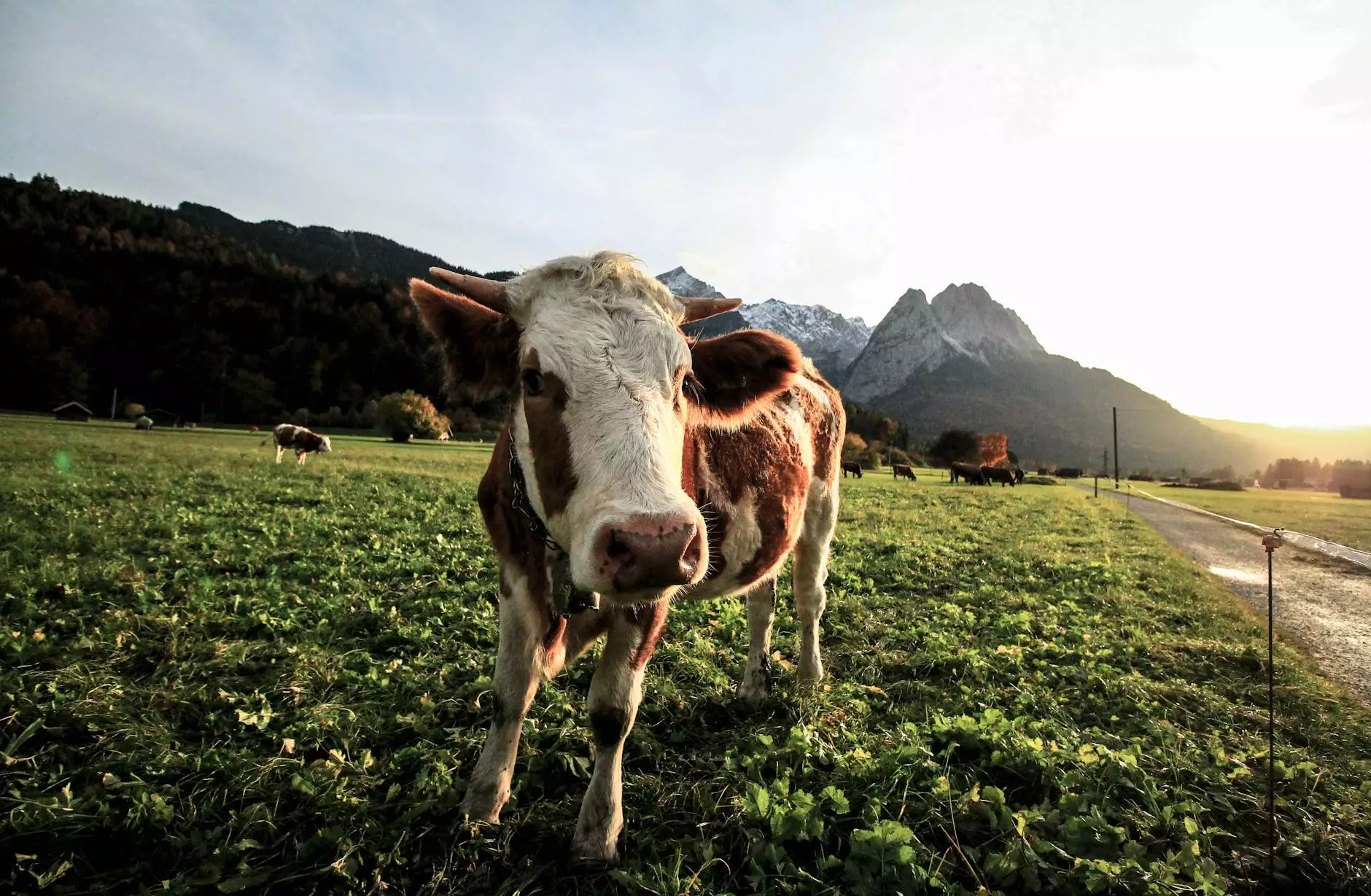
The world of agriculture is rapidly evolving, and the integration of technology is at the forefront of this transformation. One of the most promising avenues emerging from this tech-driven agricultural revolution is the use of machine learning. An agriculture dataset for machine learning is not just a collection of data; it’s a key resource that can empower farmers, agribusinesses, and researchers to make informed decisions that enhance productivity and sustainability. In this comprehensive guide, we'll explore the significance of agriculture datasets, their applications, and how they can lead to better business outcomes.
Understanding the Role of Agriculture Datasets
Agriculture datasets are collections of information gathered from various agricultural practices, environmental factors, and crop performance metrics. This data can include a vast array of variables:
- Soil quality and composition
- Weather patterns and climate data
- Crop health indicators
- Pest and disease prevalence
- Yield data and historical performances
- Market prices and economic trends
By leveraging an agriculture dataset for machine learning, stakeholders can harness the power of big data analytics to enhance decision-making processes. For instance, farmers can analyze past performances of crops using predictive algorithms, aiming to optimize the right planting strategies, irrigation practices, and fertilization schedules.
The Importance of Data in Modern Agriculture
In today’s fast-paced agricultural landscape, data is often referred to as the new oil. Here are a few key reasons why:
1. Precision Farming
Precision farming involves using detailed information on soil conditions and crop health to make decisions that maximize yield and reduce waste. An agriculture dataset for machine learning enables farmers to:
- Identify the most productive areas of their fields.
- Monitor crop health in real-time using sensors and drones.
- Optimize resource usage (water, nutrients) to minimize costs and environmental impact.
2. Predictive Analytics
Machine learning algorithms allow for predictive analysis of crop yields and market demand. By using historical data, farmers can forecast their production and pricing strategies successfully. This is crucial, especially when dealing with:
- Volatile market conditions
- Climate change effects on farming
- Supply chain challenges
3. Enhanced Sustainability
With a growing emphasis on sustainable farming, agricultural datasets help in determining the best agricultural practices that lead to reduced carbon footprints. Through data analysis, businesses can:
- Implement crop rotation systems effectively.
- Reduce chemical usage in pest control.
- Enhance biodiversity by analyzing and implementing best practices.
Applications of Agriculture Datasets in Business
The applications of an agriculture dataset for machine learning extend far beyond individual farmers. Businesses in the agricultural sector can use this data to drive significant improvements across various operations:
A. Crop Management and Yield Prediction
Through analysis of historical yield data, machine learning models can predict future crop outputs, thereby assisting businesses in:
- Planning inventory levels.
- Arranging logistics for product distribution.
- Determining the optimum times for planting and harvesting.
B. Pest and Disease Forecasting
Agricultural businesses can use data-driven models to forecast pest infestations and plant diseases. This allows for timely interventions, which can minimize crop losses significantly. Utilizing machine learning algorithms, businesses can:
- Develop integrated pest management strategies.
- Reduce the reliance on chemical pesticides.
- Enhance crop resilience through informed decision-making.
C. Market Analysis and Pricing Strategy
Understanding market trends is essential for profitability in the agricultural sector. By analyzing market data alongside environmental factors, businesses can develop effective pricing strategies. They can:
- Determine when to sell products based on predicted market conditions.
- Analyze competitor pricing strategies effectively.
- Enhance negotiation tactics using data insights.
Challenges in Utilizing Agriculture Datasets
While the possibilities with an agriculture dataset for machine learning are promising, they are not without challenges. Here are some potential issues that agribusinesses might face:
1. Data Quality and Availability
The effectiveness of machine learning relies heavily on the quality of the data. Many agricultural datasets may be incomplete, outdated, or inaccurate. It is essential to:
- Invest in technologies that ensure the accuracy of data collection.
- Partner with research organizations for high-quality datasets.
2. Technical Expertise
Successfully implementing machine learning in agriculture requires specialized skills in data science and machine learning. Organizations may need to:
- Invest in training programs for existing staff.
- Hire data scientists with experience in agricultural applications.
3. Integration with Legacy Systems
Many agricultural businesses still rely on legacy systems for daily operations. Integrating modern machine learning tools with these systems can be challenging. It may require:
- Custom development of software solutions that bridge the gap.
- Retrofitting older systems to accept new technologies.
Case Studies: Success Stories in Agriculture
Many businesses have successfully implemented machine learning using agriculture datasets, leading to impressive outcomes. Here are a couple of notable examples:
1. John Deere
John Deere utilizes machine learning algorithms to analyze data collected from smart machinery and equipment. Their platform, John Deere Operations Center, allows farmers to:
- Monitor and manage their farm from any device.
- Optimize field operations based on real-time data analytics.
- Increase yields while conserving resources.
2. Climate Corporation
The Climate Corporation provides farmers with insights based on vast amounts of data. Their FieldView platform enables farmers to make better decisions about planting and crop treatment by:
- Offering real-time weather forecasts.
- Providing yield predictions and insights based on historical performance.
- Analyzing soil conditions to recommend treatments.
The Future of Agriculture and Machine Learning
As we look to the future, the significance of an agriculture dataset for machine learning will only increase. The agricultural sector is poised to become more data-driven, leveraging technology to adapt to the challenges posed by climate change, population growth, and limited resources.
1. Innovations in AI and Robotics
The convergence of AI and robotics with agriculture datasets is creating a new wave of innovations. From autonomous tractors to drone-based surveillance, the integration of these technologies will:
- Reduce labor costs significantly.
- Enhance precision and efficiency in farming operations.
- Provide farmers with solutions that are tailored to their specific needs and conditions.
2. Continued Emphasis on Sustainability
As sustainable practices become mainstream, the role of machine learning in promoting eco-friendly solutions will be vital. Businesses will need to focus on:
- Utilizing data to enhance eco-friendliness in farming practices.
- Ensuring the long-term viability of agricultural resources.
Conclusion
In conclusion, an agriculture dataset for machine learning is an indispensable tool for modern agribusinesses. It unlocks opportunities for increased efficiency, sustainability, and profit margins. As technology continues to advance, embracing machine learning in agriculture will not just be beneficial but necessary for maintaining a competitive edge in a rapidly changing environment. Those who invest in and leverage agricultural datasets today will pave the way for a flourishing future in farming and agribusiness.